A Study of Proposed Regulatory Framework for modifications to AI/ML based SaMD
Introduction
In this article, the structure is based on FDA’s proposed Regulatory Framework for modifications to AI/ML based Software based Medical Device (SaMD). Visual organization of contents is provided, and also the comparison between AI/ML SaMD and other cleared SaMD is introduced in the related chapters.
The proposed framework can be divided into two categories, risk basis and approach. Based on the intended use and type of modifications, the corresponding risks are determined.
To balance the benefits and risks, and provide access to safe and effective AI/ML based SaMD,TPLC approach is proposed by FDA and is illustrated in Fig 3.
Although it is not finalized guidance, this framework had provided useful reference regarding the development of future guidance, and the most import, the principles how FDA evaluates the technology.
Why new total regulatory approach needed?
Before the being booming in popularity of AI/ML type SaMD , most cleared SaMD and even some AI/ML can be considered as “locked [1]” algorithm, which means algorithm will not be changed after clearance, and any change may be subjected regulatory approval including special 510K, or even traditional 510K.
However, existing reviewing process can not fit the current AI/ML SaMD, where is intensively involved modification due to continuous performance optimization.
Framework Basis
The 510K software modification guidance focuses on the risks to user/patients resulting from the software change. In this framework, it is based the internationally harmonized International Medical Device Regulators Forum (IMDRF) risk categorization principle, FDA’s benefits-risk framework, risk management principles in the software modification guidance, and the organization-based TPLC approach as envisioned in the Digital Health Software Precertification (Pre-Cert) Program.
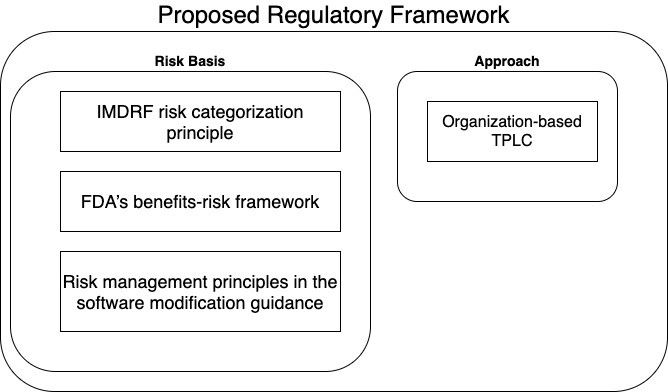
The IMDRF SaMD Risk categorization framework takes a risk-based approach to categorize SaMD based on intended use, similar to traditional risk-based approaches used by FDA. The IMDRF risk framework identifies two major factors, (1) Significance of information provided by SaMD to Healthcare decision (2) State of healthcare situation or condition, as providing a description of intended use of the SaMD.
These factors describing intended use can be used to place the AI/ML based SaMD into one of four categories, from lowest (I) to highest (IV) to reflect the risk associated the clinical situation and device use. It is depicted in Fig 2.
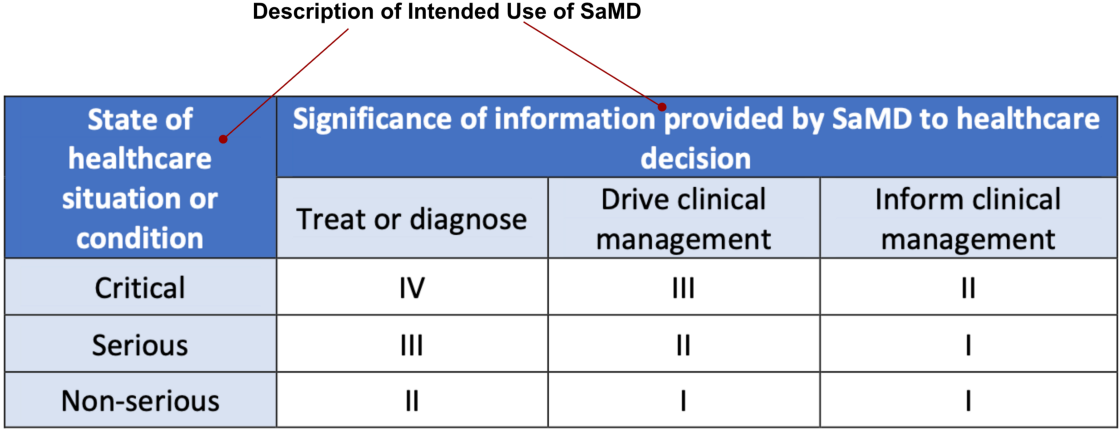
In any medical device development, the first task is to determine the intended use. Same principle is applied to AI/ML base SaMD, and it will influence the scope of SaMD Pre-specifications (SPS), which will be mentioned in the following sections.
Type of AI/ML based Modifications
Continuous modifications are expected in AI/ML based SaMD, and some modifications may not require a premarket review according to “Deciding When to Submit a 510(k) for a Software Change to an Existing Device”. But this guidance anticipates that many modifications to AI/ML based SaMD involve algorithm architecture modifications and re-training with new data sets would be subjected to premarket review.
The types of modifications generally fall into three broad categories.
Performance- Clinical and Analytical performance;
Inputs used by the algorithm and their clinical association to the SaMD output; and/or
Intended use — The intended use of the SaMD, as outlined in Fig 2 and in the IMDRF risk categorization framework, described through the significance of information provided by the SaMD for the state of the healthcare situation or condition
The changes may not be mutually exclusive, and are further grouped into followings.
i. Modifications related to the performance , with no change to the intended use or new input: For this type of modification, the manufacturer commonly aims to update the users on the performance, without changing any of the explicit use claims about their products.
ii. Modifications related to inputs, with no change to the intended use, These modifications may involve the changes to the algorithm for use with new types of input signals, but do not change the product use claims. Examples as followings. (a) Expanding the SaMD’s compatibility with other sources of the same input types. (b)Adding different input data type
iii. Modifications related to SaMD’s intended use: These modifications include those that result in a change in (1) Significance of information provided by SaMD to Healthcare decision and (2) State of healthcare situation or condition. Change related to either one may be limited in scope by the pre-specified performance objectives and algorithm change protocol.
A Total Product Lifecycle (TPLC) Regulatory Approach for AI/ML based SaMD
Applying TPLC approach to the regulation of software products is particularly important for AI/ML-base SaMD due to its ability to adapt and improve from real-world use. This approach would provide reasonable assurance of safety and effectiveness throughout the lifecycle of the organization and products so that patients, caregivers, healthcare professionals, and other users have assurance of the safety and quality of this product.
It also enables the evaluation and monitoring of a software product from its premarket development to the postmarket performance, along with continued demonstration of the organization’s excellence as Fig 3.
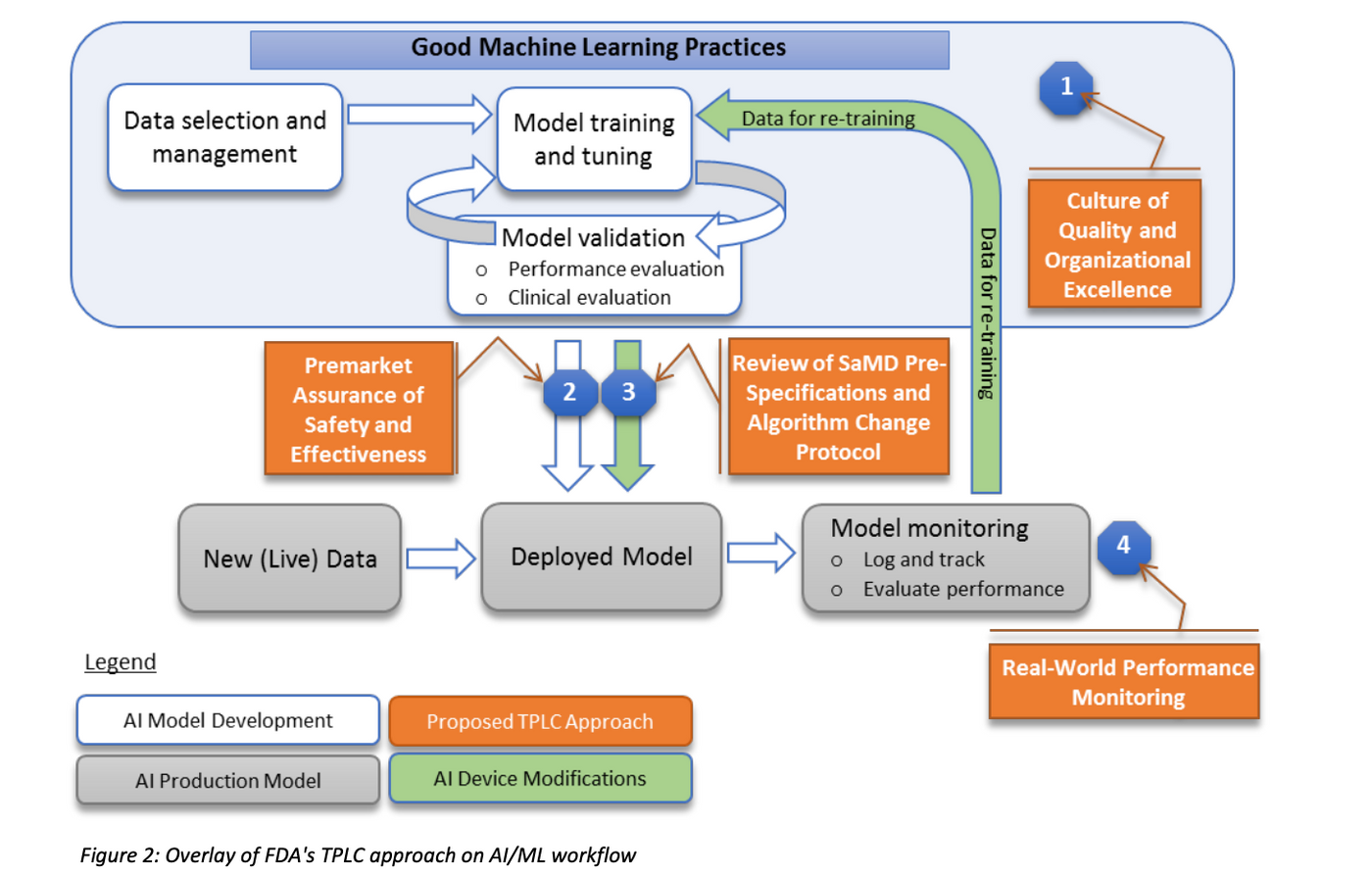
The FDA’s proposed TPLC approach is based on the following general principles that balance the benefits and risks, and provide access to safe and effective AI/ML based SaMD, as also illustrated in Fig 3.
(1) Quality system and Good Machine Learning Practices (GMLP).
For AI/ML based SaMD, FDA expects that SaMD developer embrace principles of culture of quality and organizational excellence just like the expectation to have medical device manufacturer to have an established quality system geared towards developing/delivering/maintaining high quality products throughout the lifecycle conforming appropriate standards and regulations.
AI/ML based SaMD is expected to demonstrate analytical and clinical validation, as described in the SaMD: Clinical Evaluation Guidance (Fig 4).
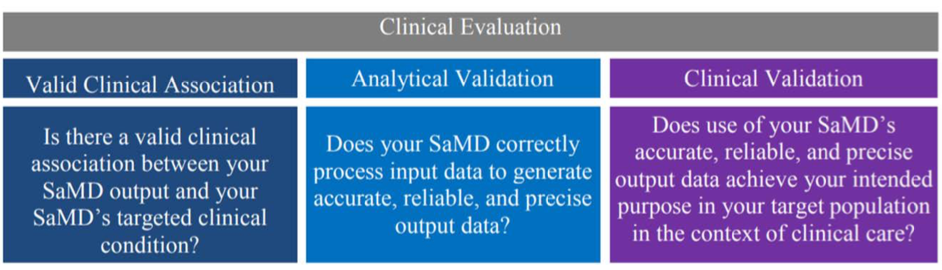
AI/ML algorithm development involves learning from data and hence prompts unique considerations that embody GMLP.
Examples of GMLP considerations as applied for SaMD include:
- Relevance of available data to the clinical problem and current clinical practice;
- Data acquired in a consistent, clinically relevant and generalizable manner that aligns with the SaMD’s intended use and modification plans;
- Appropriate separation between training, tuning, and test datasets; and
- Appropriate level of transparency (clarity) of the output and the algorithm aimed at users.
Though the proposed framework had indicated that GMLP are those AI/ML best practices (e.g., data management, feature extraction, training, and evaluation) that are akin to good software engineering practices or quality system practices, there are some difference found due to the nature of software. The suggested illustration of differences are shown in Fig 5. It is believed that GMLP must embody the existing good software engineering practice.
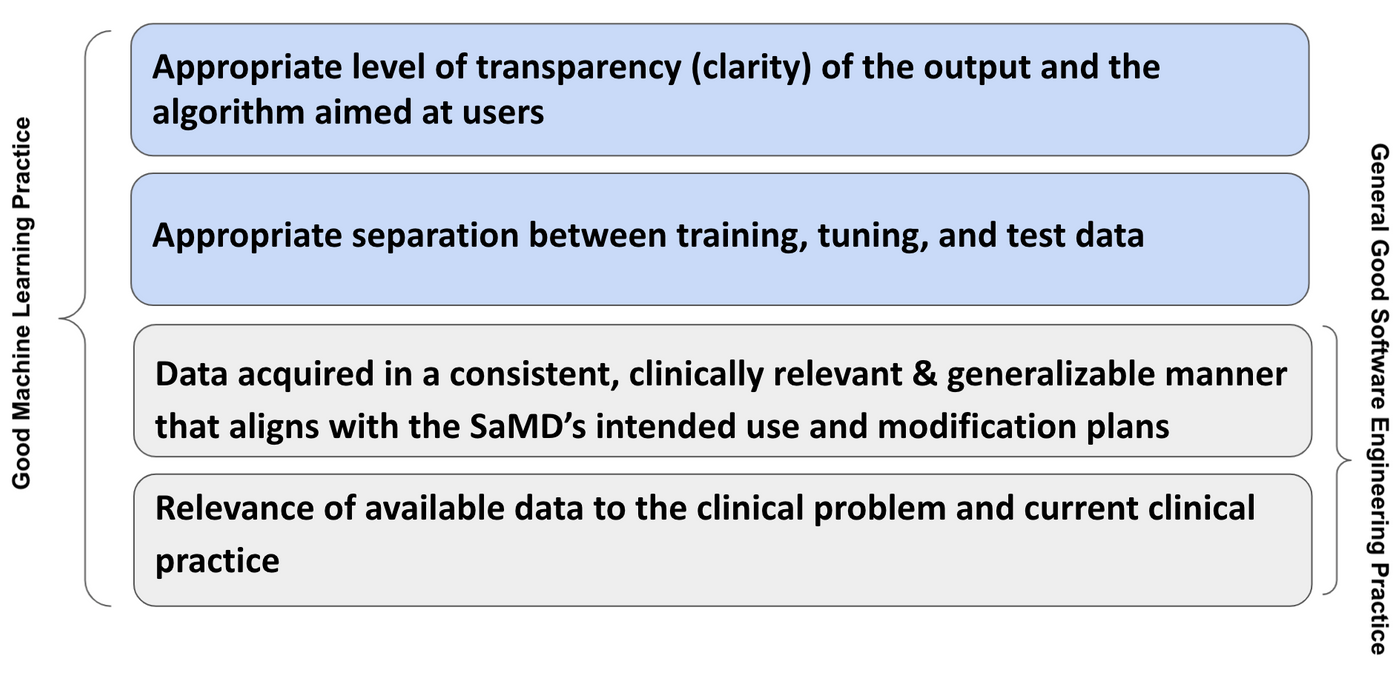
(2) Initial Premarket Assurance of Safety and Effectiveness
A framework for modifications to AI/ML-based SaMD that relies on the principle of a “predetermined change control plan” is proposed.The predetermined change control plan would include the types of anticipated modifications — SaMD Pre-Specifications (SPS)– based on the retraining and model update strategy, and the associated methodology — Algorithm Change Protocol (ACP)– being used to implement those changes in a controlled manner that manages risks to patients illustrated in Fig 6.
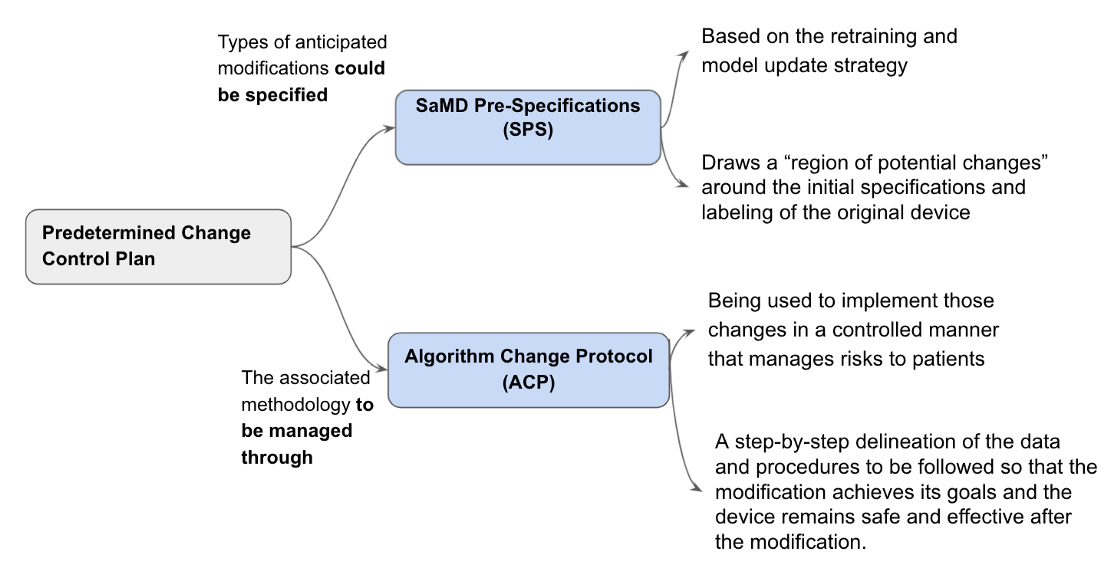
Fig 7 provides a general overview of the components of an ACP. This is “how” the algorithm will learn and change while remaining safe and effective. The components represents general good Software Engineering Practice are also indicated.
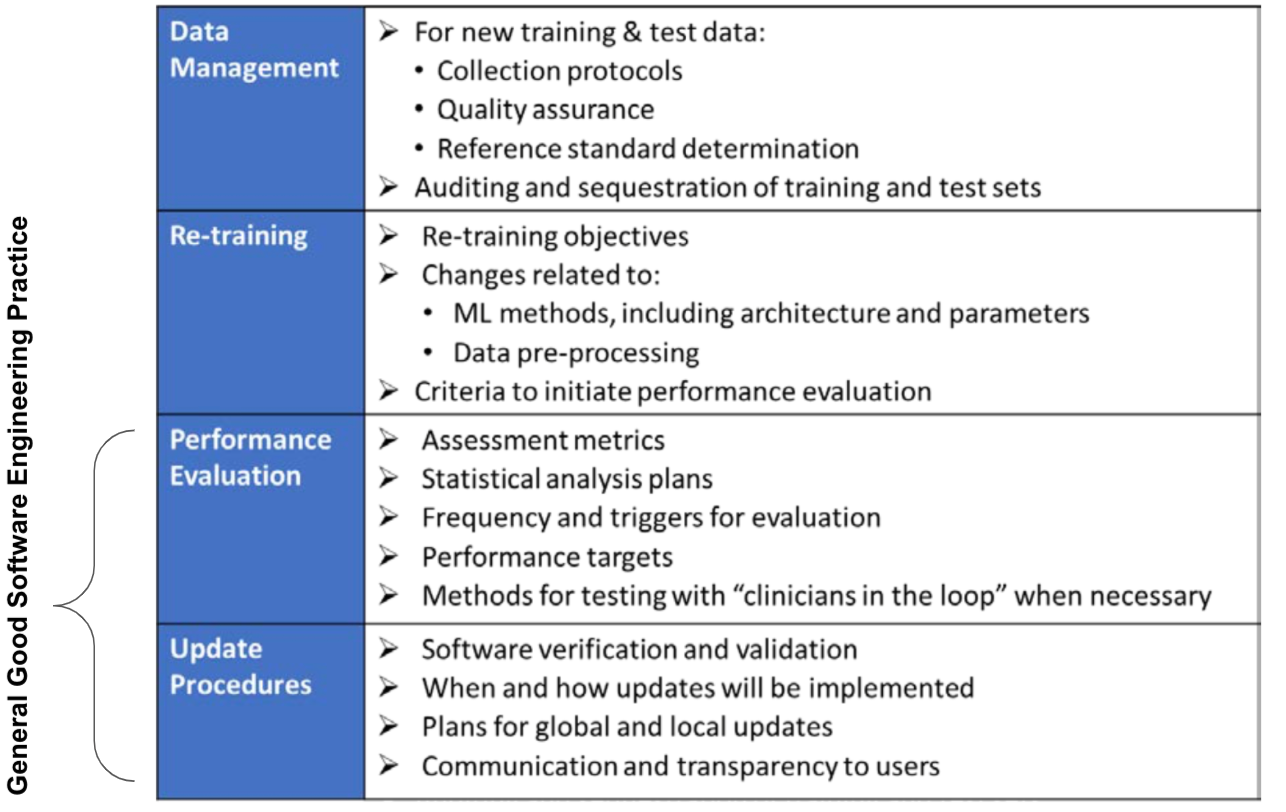
Scope and limitations for establishing SPS and ACP: The FDA acknowledges that the types of changes that could be pre-specified in a SPS and managed through an ACP may necessitate individual consideration during premarket review of benefits and risks to patients of that particular SaMD. The extent to which pre-approval of a SPS and an ACP can be relied on to support future modifications depends on various factors.
(3) Approach for modifications after initial review with an established SPS and ACP
An approach to appropriately manage risks to patients from these modifications like learning, adaptation, and optimization of AI/ML based SaMD is proposed and is outlined as Fig 8. It is noted that the flow chart should only be considered in conjunction with the accompanying text in this white paper, FDA’s proposed Regulatory Framework for modifications to AI/ML based Software based Medical Device (SaMD).
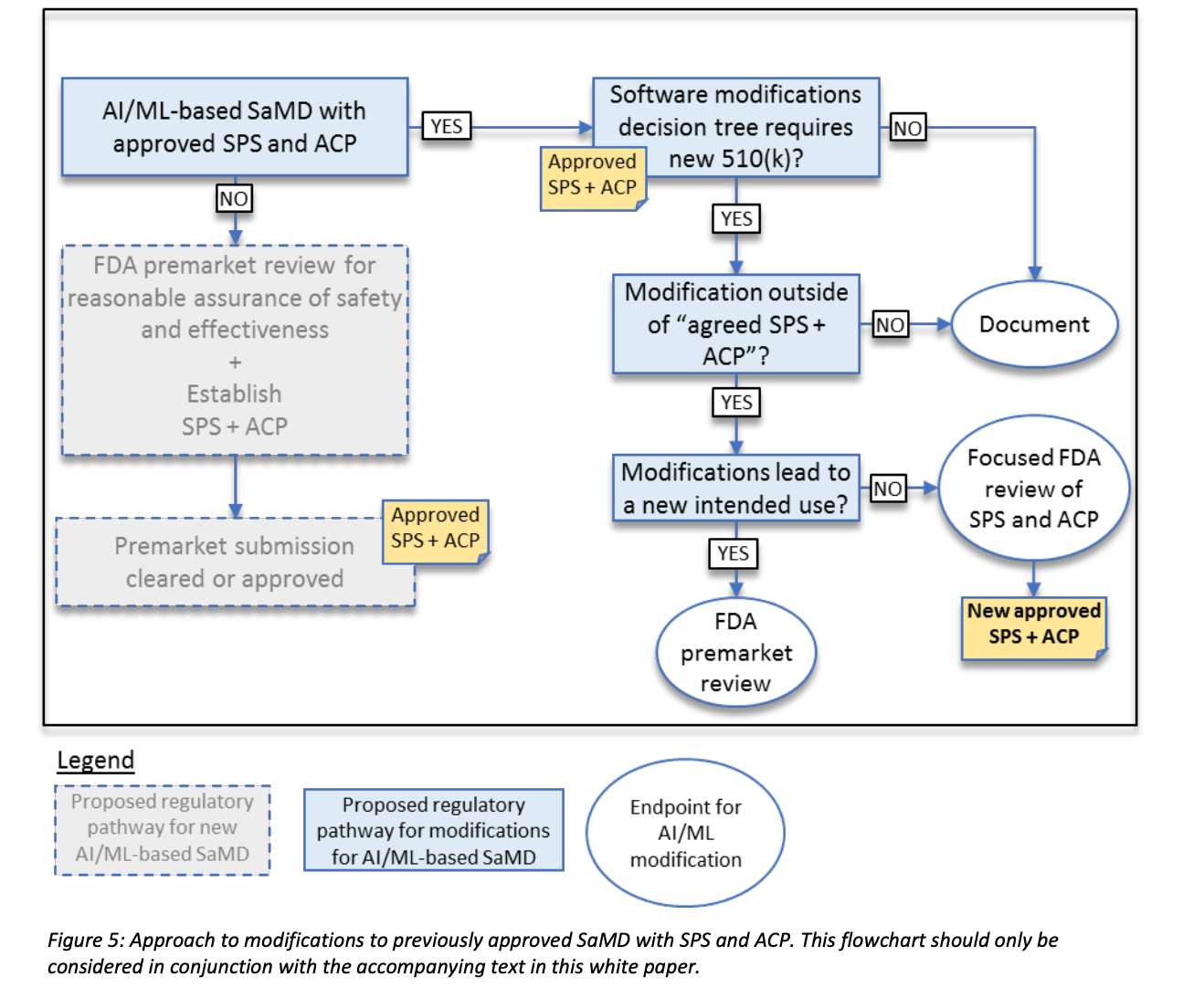
In the software modifications guidance, depending on the type of change, if the modification is beyond the intended use for which the SaMD was previously authorized, manufacturers are expected to submit a new premarket submission.
Focused Review
According to Fig 8, if the modification is with exiting intended use but outside of agreed SPS+ACP, FDA may conduct a “focused review” of the proposed SPS and ACP for a particular SaMD.
Manufacturers may leverage some of the following options to engage with FDA on the SPS and ACP for a particular SaMD shown in Fig 9.
a.Contact the appropriate review division to obtain concurrence that the modification fits under current SPS and ACP; or
b.Submit a pre-submission for a discussion on the modification and how it is within the bounds of the current SPS and ACP; or
C.Submit a premarket submission or application of the modification to SPS and ACP.
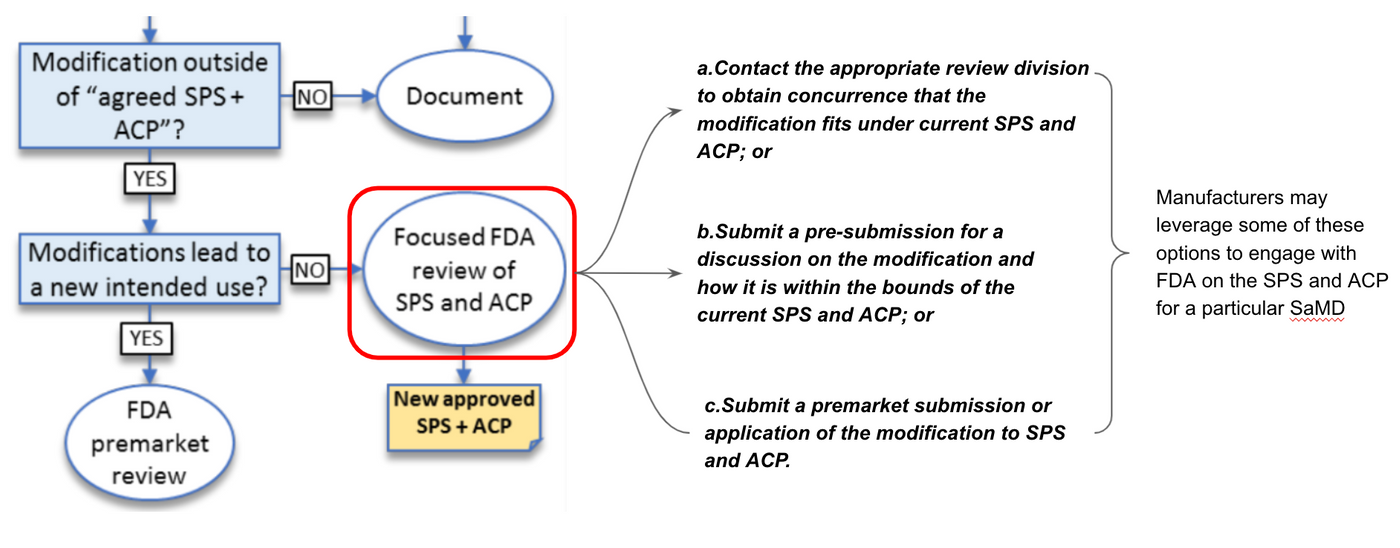
(4) Transparency and real-world performance monitoring of AI/ML based SaMD
Transparency
Transparency about the function and modifications of medical devices is a key aspect of their safety. This is especially important for devices, like SaMD that incorporate AI/ML, which change over time.
Through this framework, manufacturers would be expected to commit to the principles of transparency and real-world performance monitoring for AI/ML-based SaMD. FDA would also expect the manufacturer to provide periodic reporting to FDA on updates that were implemented as part of the approved SPS and ACP, as well as performance metrics for those SaMD. This commitment could be achieved through a variety of mechanisms.
Transparency may include updates to FDA, device companies and collaborators of the manufacturer, and the public, such as clinicians, patients, and general users.
For modifications in the SPS and ACP, manufacturers would ensure that labeling changes accurately and completely describe the modification, including its rationale, any change in inputs, and the updated performance of the SaMD. Manufacturers may also need to update the specifications or compatibility of any impacted supporting devices, accessories, or non-device components. Finally, manufacturers may consider unique mechanisms for how to be transparent — they may wish to establish communication procedures that could describe how users will be notified of updates (e.g., letters, email, software notifications) and what information could be provided (e.g., how to appropriately describe performance changes between the current and previous version).
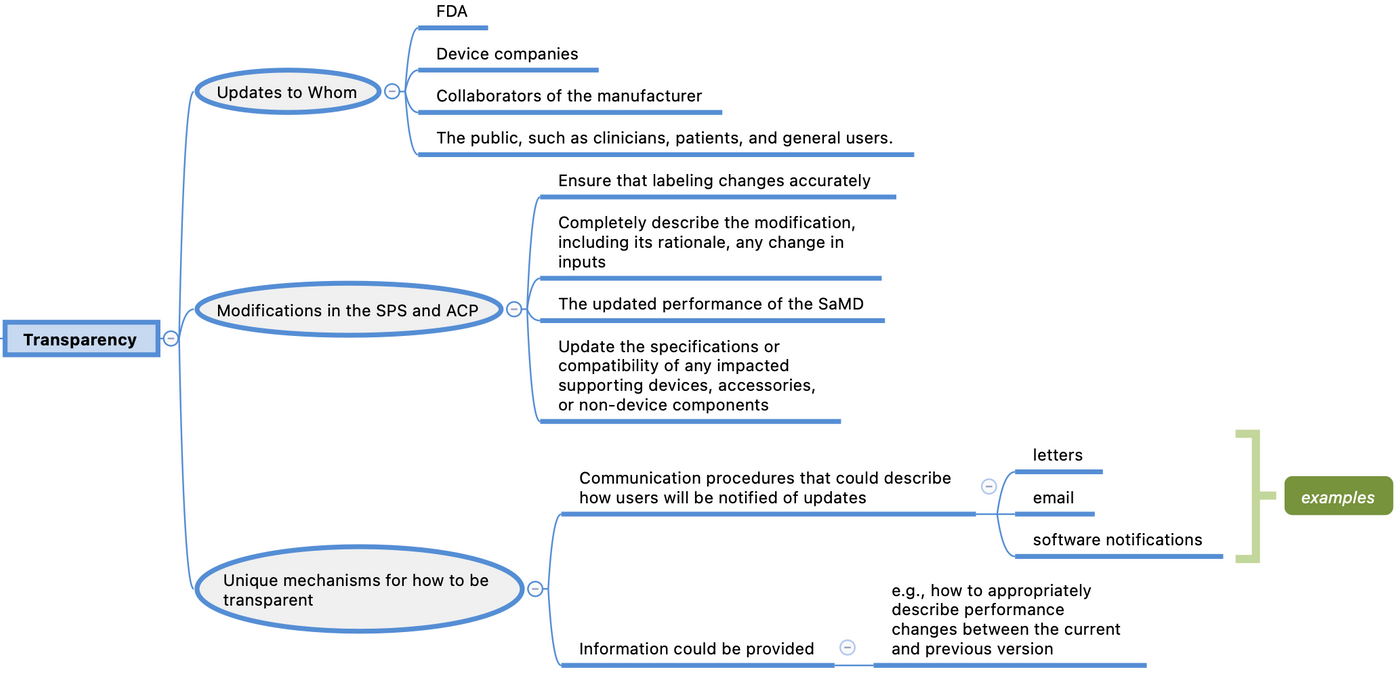
Real-world performance monitoring
Real-world performance monitoring may also be achieved in a variety of suggested mechanisms that are currently employed or under pilot at FDA, such as adding to file or an annual report, Case for Quality activities, or real-world performance analytics via the Pre-Cert Program.Reporting type and frequency may be tailored based on the risk of the device, number and types of modifications, and maturity of the algorithm (i.e., quarterly reports are unlikely to be useful if the algorithm is at a mature stage with minimal changes in performance over the quarter). Involvement in pilot programs, such as Case for Quality and the Pre-Cert Program, may also impact the reporting type and frequency given the insight into the manufacturer’s TPLC and organization. Participation in these programs could provide another avenue to support continued assurance of safety and effectiveness in development and modifications of AI/ML-based SaMD.
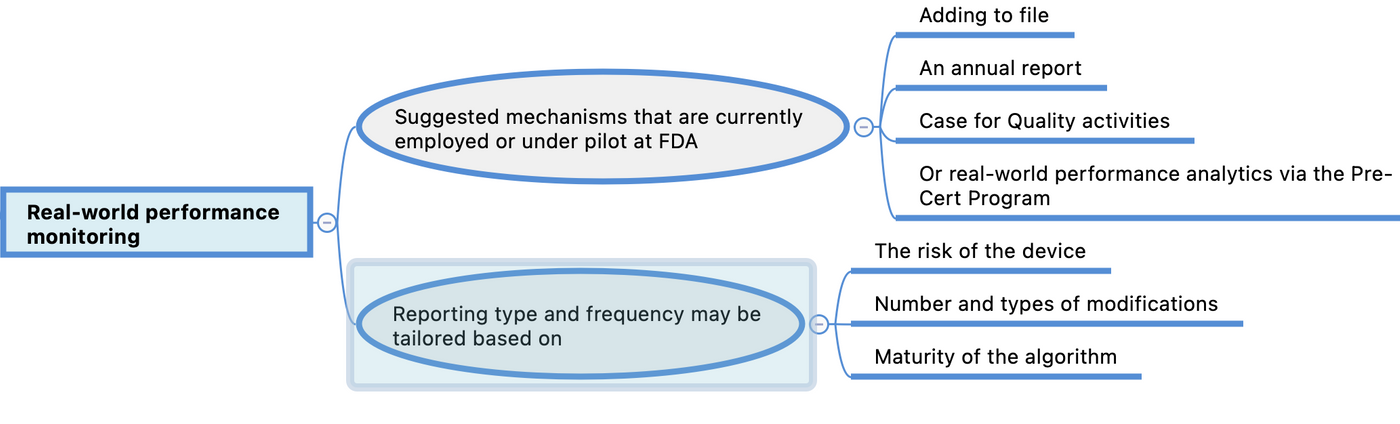
Observations
According to Fig 5 and Fig 7, traditional SaMD paradigm is essential part of AI/ML based SaMD. However, more flexibility in proposed review mechanism is found. For example, focused review is introduced instead of premarket review.
The TPLC approach had also introduce another advantage in MDR requirements, which is PMCF (Post Market Clinical Follow-UP). Such tasks can be carried out simultaneously during the performance improvement.
A visual supplemental information of TPLC approach is illustrated in Fig 12.
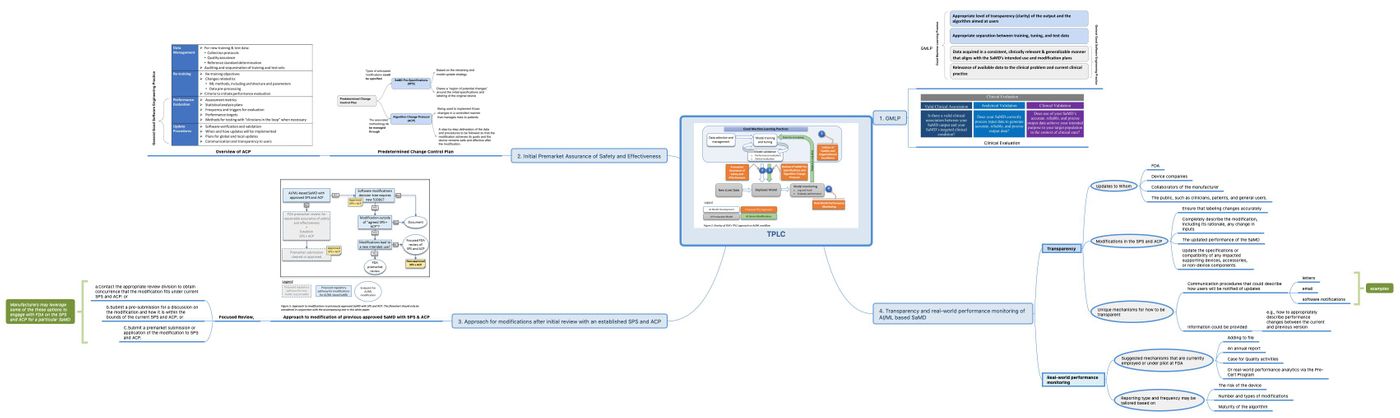
最後一張圖也可以下載放大參考。
1.FDA defines a “locked” algorithm as an algorithm that provides the same result each time the same input is applied to it and does not change with use. Examples of locked algorithms are static look-up tables, decision trees, and complex classifiers.
喜欢我的作品吗?别忘了给予支持与赞赏,让我知道在创作的路上有你陪伴,一起延续这份热忱!
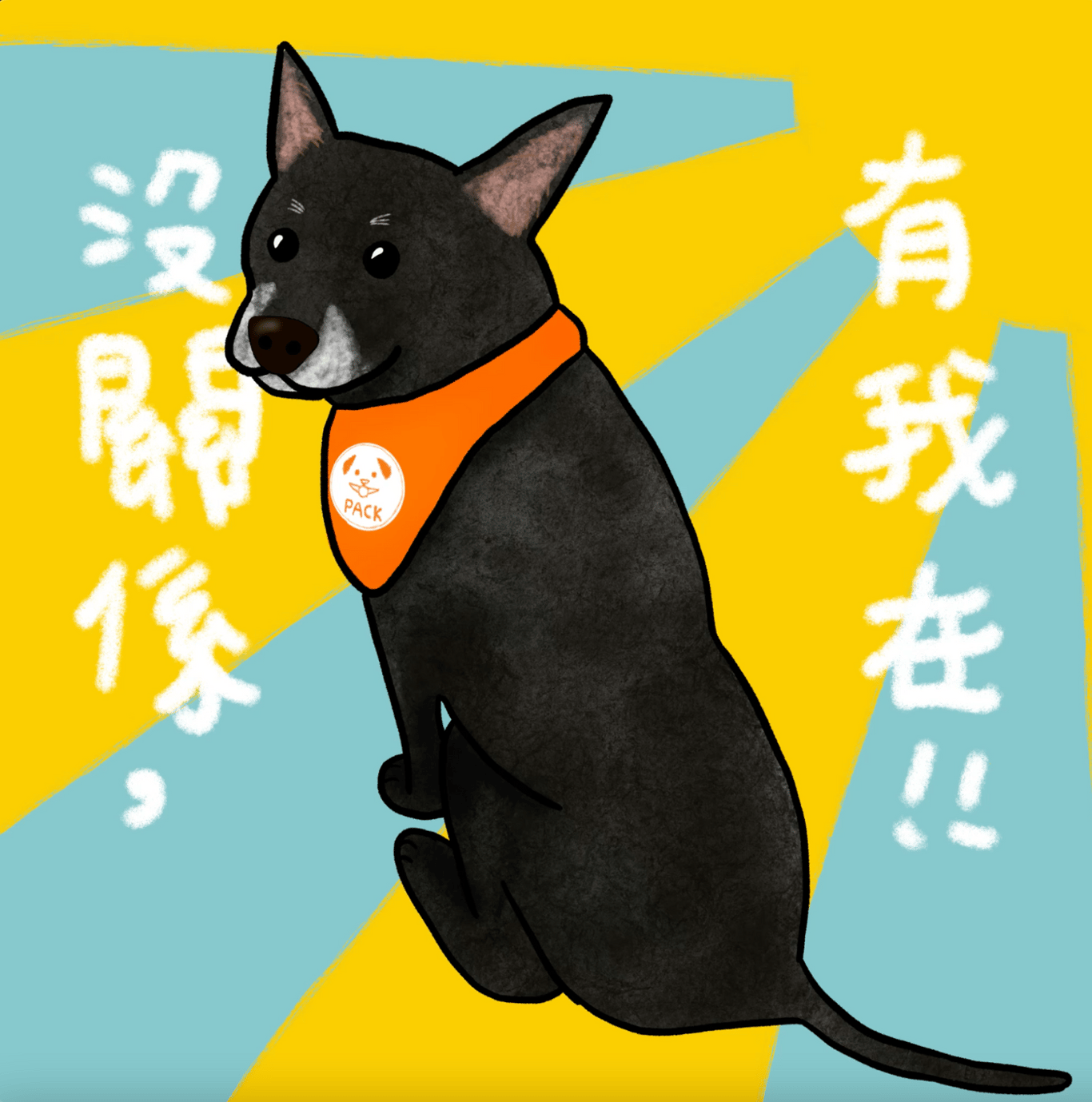
- 来自作者
- 相关推荐